Imagine a world where your devices do more than perform tasks—they make smart decisions instantly. This is the reality of Edge AI, enabled by the convergence of IoT devices, the advancement of edge computing, and recent leaps in AI technology. This blog will introduce you to the concept of Edge AI, explaining how it works and why it represents a significant shift in data processing. Join us as we unpack the essentials of Edge AI, setting the stage for a deeper understanding of this technology.
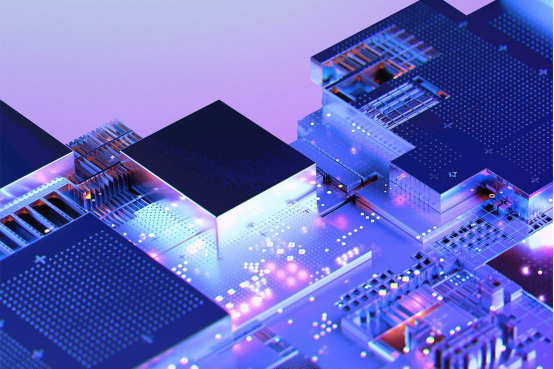
What Is Edge AI?
Before discussing what edge AI is, we need to have a basic understanding about edge computing first. Edge computing refers to the practice of processing data near the edge of the network, where the data is being generated, instead of in a centralized data processing warehouse. The “edge” in this context means literally geographical distribution, placing servers and storage closer to the location of where the data is gathered, like a factory floor, a retail store, or a home, which helps reduce latency and bandwidth use. Click here to learn more about edge computing.
Building on the concept of edge computing, Edge AI entails embedding artificial intelligence into these edge computing frameworks. Consequently, AI algorithms operate directly on devices situated at the periphery of the network, rather than relying on distant cloud servers or data centers for processing. This integration empowers devices to autonomously execute AI-driven tasks, often without needing a constant connection to central servers.
Why Integrate Artificial Intelligent into Edge Computing?
Edge computing means processing data right where it’s created, so you don’t have to send it far away for analysis. This saves time and reduces the strain on networks. When you add AI to edge computing, you supercharge the system. It not only processes data locally but also learns from it in real-time. This makes the system smart enough to make decisions, predict outcomes, and adapt on the fly. For instance, imagine a smart security camera at the edge. It doesn’t just detect motion; it can tell if it’s a person, animal, or vehicle and react accordingly. Let’s explore why AI edge computing is such a big deal.
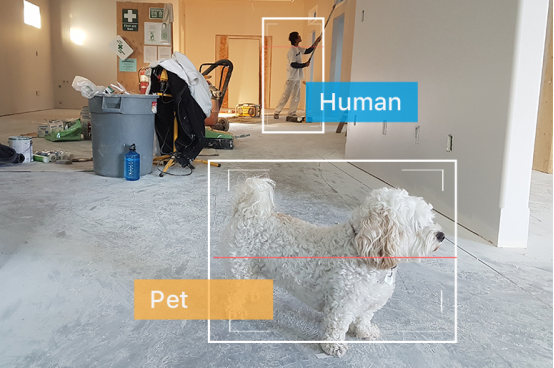
What Are the Benefits of AI Edge Computing?
AI at the edge offers several advantages over basic edge computing that does not utilize AI, including:
- Adaptive Learning: Edge AI systems can learn and adapt over time, improving their decision-making based on new data without human intervention.
- Predictive Analytics: These systems can predict outcomes and foresee potential issues before they occur, enhancing preemptive measures in scenarios like maintenance in industrial settings.
- Complex Decision-Making: AI enables more sophisticated analysis and decisions, handling complex tasks that simple automation cannot.
- Personalization: Edge AI can tailor operations and responses to the specific preferences and behaviors of users, useful in applications like personalized healthcare.
These capabilities enable Edge AI systems to perform tasks that are not only fast but also smart, providing significant added value beyond what is achievable with simple data processing at the edge.
What Are the Key Applications of Edge AI?
Now that we know Edge AI makes devices smarter, let’s see how it’s actually used in everyday life and in various industries. This will help us understand why it’s so valuable.
- Autonomous Vehicles: Edge AI processes sensory data (like images and radar) directly on the vehicle to make quick decisions about speed, direction, and obstacles. This instant processing helps the vehicle respond in real-time, enhancing safety and navigation without relying on distant servers.
- Smart Healthcare: In healthcare, wearable devices use Edge AI to continuously monitor vital signs like heart rate and blood sugar levels. The AI analyzes this data to predict potential health issues, such as heart attacks or diabetic emergencies, and can alert both the user and healthcare providers immediately.
- Industrial Automation: In factories, Edge AI enables machines to detect patterns or anomalies in their operation, predicting when parts might fail and scheduling maintenance automatically. This not only prevents downtime but also optimizes the manufacturing process by using real-time data to adjust operations.
- Smart Cities: Edge AI is used in traffic management systems to analyze traffic flow data on the spot, adjusting signal timings to reduce congestion. Similarly, in surveillance, AI can identify unusual activities or emergencies and alert authorities directly, ensuring quick response times.
- Retail: In retail settings, Edge AI helps manage inventory by tracking items in real-time and predicting stock levels. It can also offer personalized shopping experiences by analyzing customer behavior data on the spot and providing tailored recommendations.
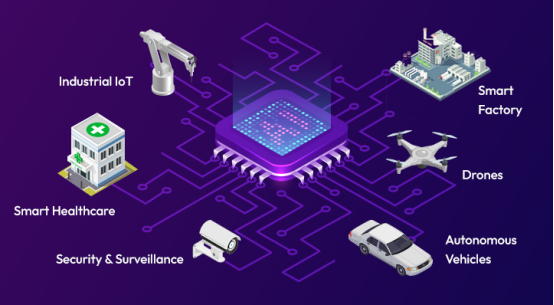
These applications show how Edge AI transforms industries by enabling devices to process data and make smart decisions locally, leading to more responsive and efficient operations.
Challenges Facing Edge AI
AI Edge computing still faces several nuanced challenges:
- Hardware Constraints: Not all edge devices are equipped with the necessary processing power. Integrating specialized AI chips that can handle sophisticated computations without consuming too much power or space is a significant engineering challenge.
- Complex Model Management: AI models require frequent updates and optimizations to maintain accuracy and efficiency. Managing these updates on potentially thousands of edge devices, each with its own operational environment and hardware capabilities, complicates deployment and ongoing maintenance.
- Data Complexity: Edge AI systems often need to process data from diverse sources and formats. Ensuring this data is accurately integrated and synchronized across multiple devices, which may not always be connected to a central network, adds layers of complexity.
- Scalability Issues: As the network of edge devices grows, ensuring that the system can scale effectively while maintaining performance is a challenge. Furthermore, interoperability among different manufacturers’ devices and platforms needs standardized protocols to allow seamless communication and function.
Addressing these challenges is crucial for the successful implementation and scalability of Edge AI solutions across various industries.
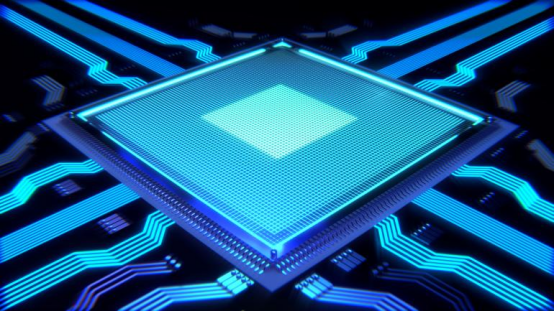
Introducing Geniatech’s Diverse AI Solutions
Geniatech’s offerings encompass a broad array of AI solutions tailored to meet the demands of modern industrial and edge computing environments. Here’s a detailed look at its product lineup and the innovative technologies it incorporates:
Single Board Computers (SBCs)
Geniatech Single Board Computers are compact and robust, designed to handle AI tasks efficiently at the edge of networks. These boards are particularly beneficial for applications requiring real-time data processing, such as IoT devices, automation systems, and smart security solutions.
System on Modules (SOMs)
Geniatech System on Modules, based on ARM and RISC-V architectures, are key to Geniatech’s strategy for modular, scalable AI solutions. They pack significant processing power into a small package, making them ideal for embedding into complex systems where space is at a premium but high performance is required.
Embedded Box PCs
Durable and versatile, Geniatech Embedded Box PCs are engineered to operate reliably in tough industrial environments. They support a wide range of applications, from manufacturing process control to advanced analytics at the data source.
AI Acceleration and Collaboration
The integration of the Hailo AI Acceleration Module enhances overall AI algorithm performance, making Geniatech’s devices quicker and more efficient at data handling. Moreover, the collaboration with NVIDIA, potentially through incorporating NVIDIA Jetson Nano™ technologies, brings superior graphics and AI processing capabilities to Geniatech AI solutions, particularly beneficial for graphics-intensive AI applications.
The Future of Edge AI Computing
Edge AI is really changing things up in areas like cars, healthcare, and factories. By handling data right where it’s collected, these devices don’t have to wait around for cloud servers, making everything faster and smarter. We’re looking at a future where these technologies not only respond quicker but also manage energy better and get smarter on their own. This means we can expect smoother and more personalized tech experiences soon.